Dominant Strategy : Buy
Time series to forecast n: 13 Jun 2023 for 4 Weeks
Methodology : Modular Neural Network (Market Volatility Analysis)
Abstract
Fresh Tracks Therapeutics Inc. Common Stock prediction model is evaluated with Modular Neural Network (Market Volatility Analysis) and Logistic Regression1,2,3,4 and it is concluded that the FRTX stock is predictable in the short/long term. Modular neural networks (MNNs) are a type of artificial neural network that can be used for market volatility analysis. MNNs are made up of multiple smaller neural networks, called modules. Each module is responsible for learning a specific task, such as identifying patterns in data or predicting future price movements. The modules are then combined to form a single neural network that can perform multiple tasks.In the context of market volatility analysis, MNNs can be used to identify patterns in market data that suggest that the market is becoming more or less volatile. This information can then be used to make predictions about future price movements. According to price forecasts for 4 Weeks period, the dominant strategy among neural network is: Buy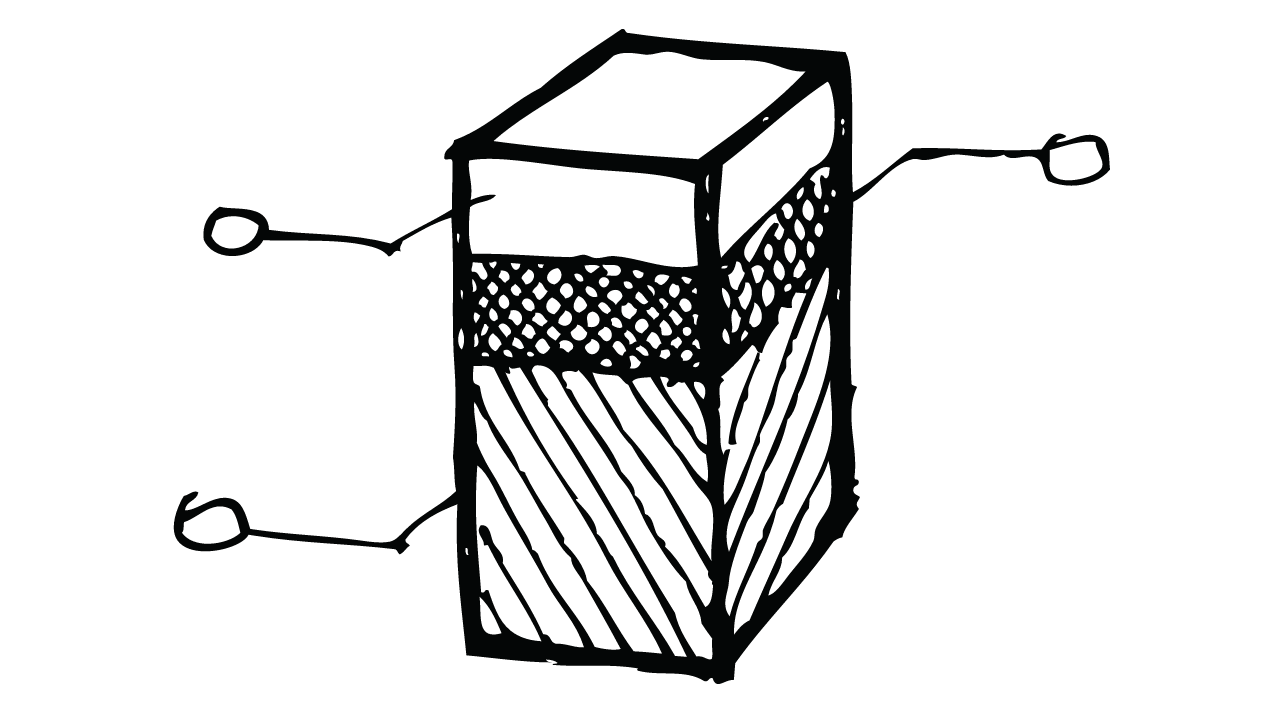
Key Points
- Market Signals
- What is prediction model?
- Can machine learning predict?
FRTX Target Price Prediction Modeling Methodology
We consider Fresh Tracks Therapeutics Inc. Common Stock Decision Process with Modular Neural Network (Market Volatility Analysis) where A is the set of discrete actions of FRTX stock holders, F is the set of discrete states, P : S × F × S → R is the transition probability distribution, R : S × F → R is the reaction function, and γ ∈ [0, 1] is a move factor for expectation.1,2,3,4
F(Logistic Regression)5,6,7= X R(Modular Neural Network (Market Volatility Analysis)) X S(n):→ 4 Weeks
n:Time series to forecast
p:Price signals of FRTX stock
j:Nash equilibria (Neural Network)
k:Dominated move
a:Best response for target price
Modular Neural Network (Market Volatility Analysis)
Modular neural networks (MNNs) are a type of artificial neural network that can be used for market volatility analysis. MNNs are made up of multiple smaller neural networks, called modules. Each module is responsible for learning a specific task, such as identifying patterns in data or predicting future price movements. The modules are then combined to form a single neural network that can perform multiple tasks.In the context of market volatility analysis, MNNs can be used to identify patterns in market data that suggest that the market is becoming more or less volatile. This information can then be used to make predictions about future price movements.Logistic Regression
In statistics, logistic regression is a type of regression analysis used when the dependent variable is categorical. Logistic regression is a probability model that predicts the probability of an event occurring based on a set of independent variables. In logistic regression, the dependent variable is represented as a binary variable, such as "yes" or "no," "true" or "false," or "sick" or "healthy." The independent variables can be continuous or categorical variables.
For further technical information as per how our model work we invite you to visit the article below:
How do AC Investment Research machine learning (predictive) algorithms actually work?
FRTX Stock Forecast (Buy or Sell) for 4 Weeks
Sample Set: Neural NetworkStock/Index: FRTX Fresh Tracks Therapeutics Inc. Common Stock
Time series to forecast n: 13 Jun 2023 for 4 Weeks
According to price forecasts for 4 Weeks period, the dominant strategy among neural network is: Buy
X axis: *Likelihood% (The higher the percentage value, the more likely the event will occur.)
Y axis: *Potential Impact% (The higher the percentage value, the more likely the price will deviate.)
Z axis (Grey to Black): *Technical Analysis%
IFRS Reconciliation Adjustments for Fresh Tracks Therapeutics Inc. Common Stock
- An entity is not required to restate prior periods to reflect the application of these amendments. The entity may restate prior periods only if it is possible to do so without the use of hindsight. If an entity restates prior periods, the restated financial statements must reflect all the requirements in this Standard for the affected financial instruments. If an entity does not restate prior periods, the entity shall recognise any difference between the previous carrying amount and the carrying amount at the beginning of the annual reporting period that includes the date of initial application of these amendments in the opening retained earnings (or other component of equity, as appropriate) of the annual reporting period that includes the date of initial application of these amendments.
- If an entity has applied paragraph 7.2.6 then at the date of initial application the entity shall recognise any difference between the fair value of the entire hybrid contract at the date of initial application and the sum of the fair values of the components of the hybrid contract at the date of initial application in the opening retained earnings (or other component of equity, as appropriate) of the reporting period that includes the date of initial application.
- For the purposes of the transition provisions in paragraphs 7.2.1, 7.2.3–7.2.28 and 7.3.2, the date of initial application is the date when an entity first applies those requirements of this Standard and must be the beginning of a reporting period after the issue of this Standard. Depending on the entity's chosen approach to applying IFRS 9, the transition can involve one or more than one date of initial application for different requirements.
- The expected credit losses on a loan commitment shall be discounted using the effective interest rate, or an approximation thereof, that will be applied when recognising the financial asset resulting from the loan commitment. This is because for the purpose of applying the impairment requirements, a financial asset that is recognised following a draw down on a loan commitment shall be treated as a continuation of that commitment instead of as a new financial instrument. The expected credit losses on the financial asset shall therefore be measured considering the initial credit risk of the loan commitment from the date that the entity became a party to the irrevocable commitment.
*International Financial Reporting Standards (IFRS) adjustment process involves reviewing the company's financial statements and identifying any differences between the company's current accounting practices and the requirements of the IFRS. If there are any such differences, neural network makes adjustments to financial statements to bring them into compliance with the IFRS.
Conclusions
Fresh Tracks Therapeutics Inc. Common Stock is assigned short-term Ba1 & long-term Ba1 estimated rating. Fresh Tracks Therapeutics Inc. Common Stock prediction model is evaluated with Modular Neural Network (Market Volatility Analysis) and Logistic Regression1,2,3,4 and it is concluded that the FRTX stock is predictable in the short/long term. According to price forecasts for 4 Weeks period, the dominant strategy among neural network is: Buy
FRTX Fresh Tracks Therapeutics Inc. Common Stock Financial Analysis*
Rating | Short-Term | Long-Term Senior |
---|---|---|
Outlook* | Ba1 | Ba1 |
Income Statement | Baa2 | Baa2 |
Balance Sheet | Caa2 | B1 |
Leverage Ratios | Ba1 | B1 |
Cash Flow | Baa2 | Baa2 |
Rates of Return and Profitability | Ba3 | Caa2 |
*Financial analysis is the process of evaluating a company's financial performance and position by neural network. It involves reviewing the company's financial statements, including the balance sheet, income statement, and cash flow statement, as well as other financial reports and documents.
How does neural network examine financial reports and understand financial state of the company?
Prediction Confidence Score
References
- Hartford J, Lewis G, Taddy M. 2016. Counterfactual prediction with deep instrumental variables networks. arXiv:1612.09596 [stat.AP]
- Bengio Y, Schwenk H, Senécal JS, Morin F, Gauvain JL. 2006. Neural probabilistic language models. In Innovations in Machine Learning: Theory and Applications, ed. DE Holmes, pp. 137–86. Berlin: Springer
- Dudik M, Erhan D, Langford J, Li L. 2014. Doubly robust policy evaluation and optimization. Stat. Sci. 29:485–511
- Bai J, Ng S. 2002. Determining the number of factors in approximate factor models. Econometrica 70:191–221
- Bickel P, Klaassen C, Ritov Y, Wellner J. 1998. Efficient and Adaptive Estimation for Semiparametric Models. Berlin: Springer
- Dudik M, Langford J, Li L. 2011. Doubly robust policy evaluation and learning. In Proceedings of the 28th International Conference on Machine Learning, pp. 1097–104. La Jolla, CA: Int. Mach. Learn. Soc.
- Bastani H, Bayati M. 2015. Online decision-making with high-dimensional covariates. Work. Pap., Univ. Penn./ Stanford Grad. School Bus., Philadelphia/Stanford, CA
Frequently Asked Questions
Q: What is the prediction methodology for FRTX stock?A: FRTX stock prediction methodology: We evaluate the prediction models Modular Neural Network (Market Volatility Analysis) and Logistic Regression
Q: Is FRTX stock a buy or sell?
A: The dominant strategy among neural network is to Buy FRTX Stock.
Q: Is Fresh Tracks Therapeutics Inc. Common Stock stock a good investment?
A: The consensus rating for Fresh Tracks Therapeutics Inc. Common Stock is Buy and is assigned short-term Ba1 & long-term Ba1 estimated rating.
Q: What is the consensus rating of FRTX stock?
A: The consensus rating for FRTX is Buy.
Q: What is the prediction period for FRTX stock?
A: The prediction period for FRTX is 4 Weeks