Dominant Strategy : Hold
Time series to forecast n: 13 Jun 2023 for 6 Month
Methodology : Modular Neural Network (Social Media Sentiment Analysis)
Abstract
Equitable Holdings Inc. Depositary Shares prediction model is evaluated with Modular Neural Network (Social Media Sentiment Analysis) and Pearson Correlation1,2,3,4 and it is concluded that the EQH^A stock is predictable in the short/long term. A modular neural network (MNN) is a type of artificial neural network that can be used for social media sentiment analysis. MNNs are made up of multiple smaller neural networks, called modules. Each module is responsible for learning a specific task, such as identifying sentiment in text or identifying patterns in data. The modules are then combined to form a single neural network that can perform multiple tasks. In the context of social media sentiment analysis, MNNs can be used to identify the sentiment of social media posts, such as tweets, Facebook posts, and Instagram stories. This information can then be used to filter out irrelevant or unwanted content, to identify trends in public opinion, and to target users with relevant advertising. According to price forecasts for 6 Month period, the dominant strategy among neural network is: Hold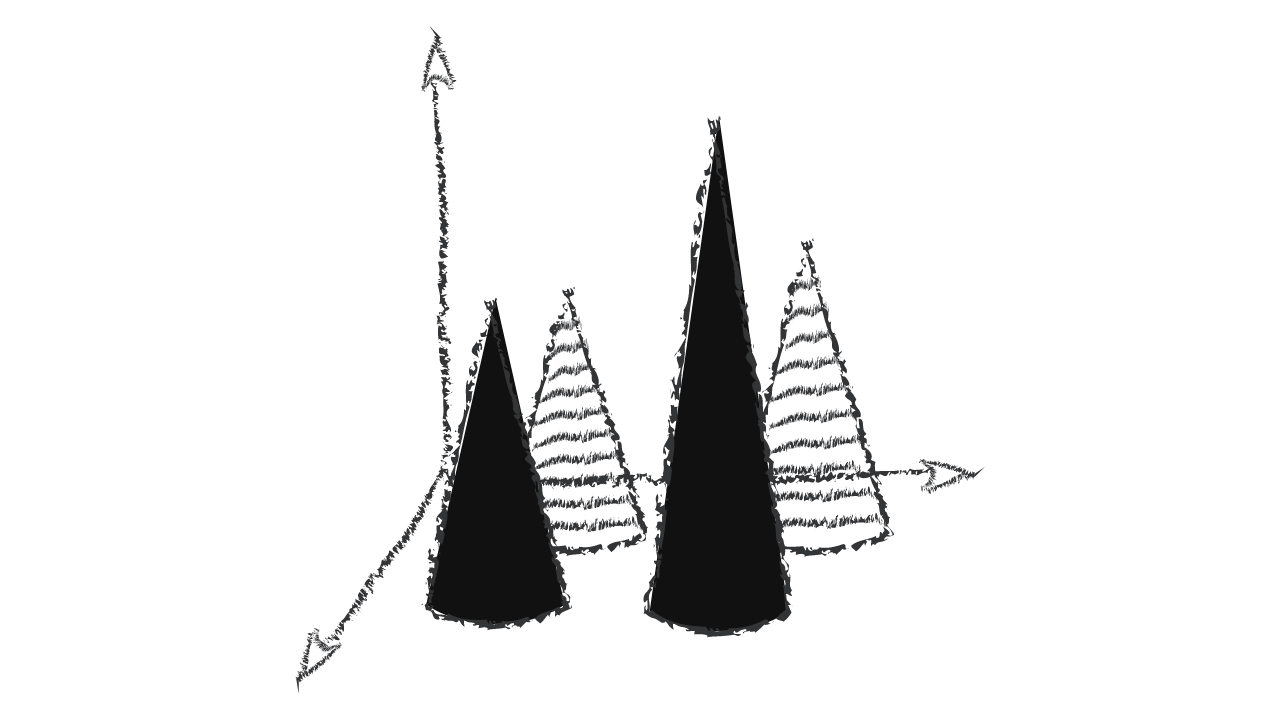
Key Points
- How do you pick a stock?
- How can neural networks improve predictions?
- Is Target price a good indicator?
EQH^A Target Price Prediction Modeling Methodology
We consider Equitable Holdings Inc. Depositary Shares Decision Process with Modular Neural Network (Social Media Sentiment Analysis) where A is the set of discrete actions of EQH^A stock holders, F is the set of discrete states, P : S × F × S → R is the transition probability distribution, R : S × F → R is the reaction function, and γ ∈ [0, 1] is a move factor for expectation.1,2,3,4
F(Pearson Correlation)5,6,7= X R(Modular Neural Network (Social Media Sentiment Analysis)) X S(n):→ 6 Month
n:Time series to forecast
p:Price signals of EQH^A stock
j:Nash equilibria (Neural Network)
k:Dominated move
a:Best response for target price
Modular Neural Network (Social Media Sentiment Analysis)
A modular neural network (MNN) is a type of artificial neural network that can be used for social media sentiment analysis. MNNs are made up of multiple smaller neural networks, called modules. Each module is responsible for learning a specific task, such as identifying sentiment in text or identifying patterns in data. The modules are then combined to form a single neural network that can perform multiple tasks. In the context of social media sentiment analysis, MNNs can be used to identify the sentiment of social media posts, such as tweets, Facebook posts, and Instagram stories. This information can then be used to filter out irrelevant or unwanted content, to identify trends in public opinion, and to target users with relevant advertising.Pearson Correlation
Pearson correlation, also known as Pearson's product-moment correlation, is a measure of the linear relationship between two variables. It is a statistical measure that assesses the strength and direction of a linear relationship between two variables. The sign of the correlation coefficient indicates the direction of the relationship, while the magnitude of the correlation coefficient indicates the strength of the relationship. A correlation coefficient of 0.9 indicates a strong positive correlation, while a correlation coefficient of 0.2 indicates a weak positive correlation.
For further technical information as per how our model work we invite you to visit the article below:
How do AC Investment Research machine learning (predictive) algorithms actually work?
EQH^A Stock Forecast (Buy or Sell) for 6 Month
Sample Set: Neural NetworkStock/Index: EQH^A Equitable Holdings Inc. Depositary Shares
Time series to forecast n: 13 Jun 2023 for 6 Month
According to price forecasts for 6 Month period, the dominant strategy among neural network is: Hold
X axis: *Likelihood% (The higher the percentage value, the more likely the event will occur.)
Y axis: *Potential Impact% (The higher the percentage value, the more likely the price will deviate.)
Z axis (Grey to Black): *Technical Analysis%
IFRS Reconciliation Adjustments for Equitable Holdings Inc. Depositary Shares
- If there are changes in circumstances that affect hedge effectiveness, an entity may have to change the method for assessing whether a hedging relationship meets the hedge effectiveness requirements in order to ensure that the relevant characteristics of the hedging relationship, including the sources of hedge ineffectiveness, are still captured.
- Lifetime expected credit losses are generally expected to be recognised before a financial instrument becomes past due. Typically, credit risk increases significantly before a financial instrument becomes past due or other lagging borrower-specific factors (for example, a modification or restructuring) are observed. Consequently when reasonable and supportable information that is more forward-looking than past due information is available without undue cost or effort, it must be used to assess changes in credit risk.
- Time value of money is the element of interest that provides consideration for only the passage of time. That is, the time value of money element does not provide consideration for other risks or costs associated with holding the financial asset. In order to assess whether the element provides consideration for only the passage of time, an entity applies judgement and considers relevant factors such as the currency in which the financial asset is denominated and the period for which the interest rate is set.
- Expected credit losses reflect an entity's own expectations of credit losses. However, when considering all reasonable and supportable information that is available without undue cost or effort in estimating expected credit losses, an entity should also consider observable market information about the credit risk of the particular financial instrument or similar financial instruments.
*International Financial Reporting Standards (IFRS) adjustment process involves reviewing the company's financial statements and identifying any differences between the company's current accounting practices and the requirements of the IFRS. If there are any such differences, neural network makes adjustments to financial statements to bring them into compliance with the IFRS.
Conclusions
Equitable Holdings Inc. Depositary Shares is assigned short-term Ba1 & long-term Ba1 estimated rating. Equitable Holdings Inc. Depositary Shares prediction model is evaluated with Modular Neural Network (Social Media Sentiment Analysis) and Pearson Correlation1,2,3,4 and it is concluded that the EQH^A stock is predictable in the short/long term. According to price forecasts for 6 Month period, the dominant strategy among neural network is: Hold
EQH^A Equitable Holdings Inc. Depositary Shares Financial Analysis*
Rating | Short-Term | Long-Term Senior |
---|---|---|
Outlook* | Ba1 | Ba1 |
Income Statement | Baa2 | B3 |
Balance Sheet | B2 | Caa2 |
Leverage Ratios | Caa2 | B2 |
Cash Flow | Ba2 | Baa2 |
Rates of Return and Profitability | Caa2 | Baa2 |
*Financial analysis is the process of evaluating a company's financial performance and position by neural network. It involves reviewing the company's financial statements, including the balance sheet, income statement, and cash flow statement, as well as other financial reports and documents.
How does neural network examine financial reports and understand financial state of the company?
Prediction Confidence Score
References
- Matzkin RL. 2007. Nonparametric identification. In Handbook of Econometrics, Vol. 6B, ed. J Heckman, E Learner, pp. 5307–68. Amsterdam: Elsevier
- Hartigan JA, Wong MA. 1979. Algorithm as 136: a k-means clustering algorithm. J. R. Stat. Soc. Ser. C 28:100–8
- Van der Vaart AW. 2000. Asymptotic Statistics. Cambridge, UK: Cambridge Univ. Press
- Nie X, Wager S. 2019. Quasi-oracle estimation of heterogeneous treatment effects. arXiv:1712.04912 [stat.ML]
- Wager S, Athey S. 2017. Estimation and inference of heterogeneous treatment effects using random forests. J. Am. Stat. Assoc. 113:1228–42
- Greene WH. 2000. Econometric Analysis. Upper Saddle River, N J: Prentice Hall. 4th ed.
- Ashley, R. (1988), "On the relative worth of recent macroeconomic forecasts," International Journal of Forecasting, 4, 363–376.
Frequently Asked Questions
Q: What is the prediction methodology for EQH^A stock?A: EQH^A stock prediction methodology: We evaluate the prediction models Modular Neural Network (Social Media Sentiment Analysis) and Pearson Correlation
Q: Is EQH^A stock a buy or sell?
A: The dominant strategy among neural network is to Hold EQH^A Stock.
Q: Is Equitable Holdings Inc. Depositary Shares stock a good investment?
A: The consensus rating for Equitable Holdings Inc. Depositary Shares is Hold and is assigned short-term Ba1 & long-term Ba1 estimated rating.
Q: What is the consensus rating of EQH^A stock?
A: The consensus rating for EQH^A is Hold.
Q: What is the prediction period for EQH^A stock?
A: The prediction period for EQH^A is 6 Month