Dominant Strategy : Buy
Time series to forecast n: 17 Jun 2023 for 1 Year
Methodology : Modular Neural Network (Market News Sentiment Analysis)
Abstract
Bear Creek Mining Corporation prediction model is evaluated with Modular Neural Network (Market News Sentiment Analysis) and Paired T-Test1,2,3,4 and it is concluded that the BCM:TSXV stock is predictable in the short/long term. A modular neural network (MNN) is a type of artificial neural network that can be used for news feed sentiment analysis. MNNs are made up of multiple smaller neural networks, called modules. Each module is responsible for learning a specific task, such as identifying sentiment in text or identifying patterns in data. The modules are then combined to form a single neural network that can perform multiple tasks. In the context of news feed sentiment analysis, MNNs can be used to identify the sentiment of news articles, social media posts, and other forms of online content. This information can then be used to filter out irrelevant or unwanted content, to identify trends in public opinion, and to target users with relevant advertising. According to price forecasts for 1 Year period, the dominant strategy among neural network is: Buy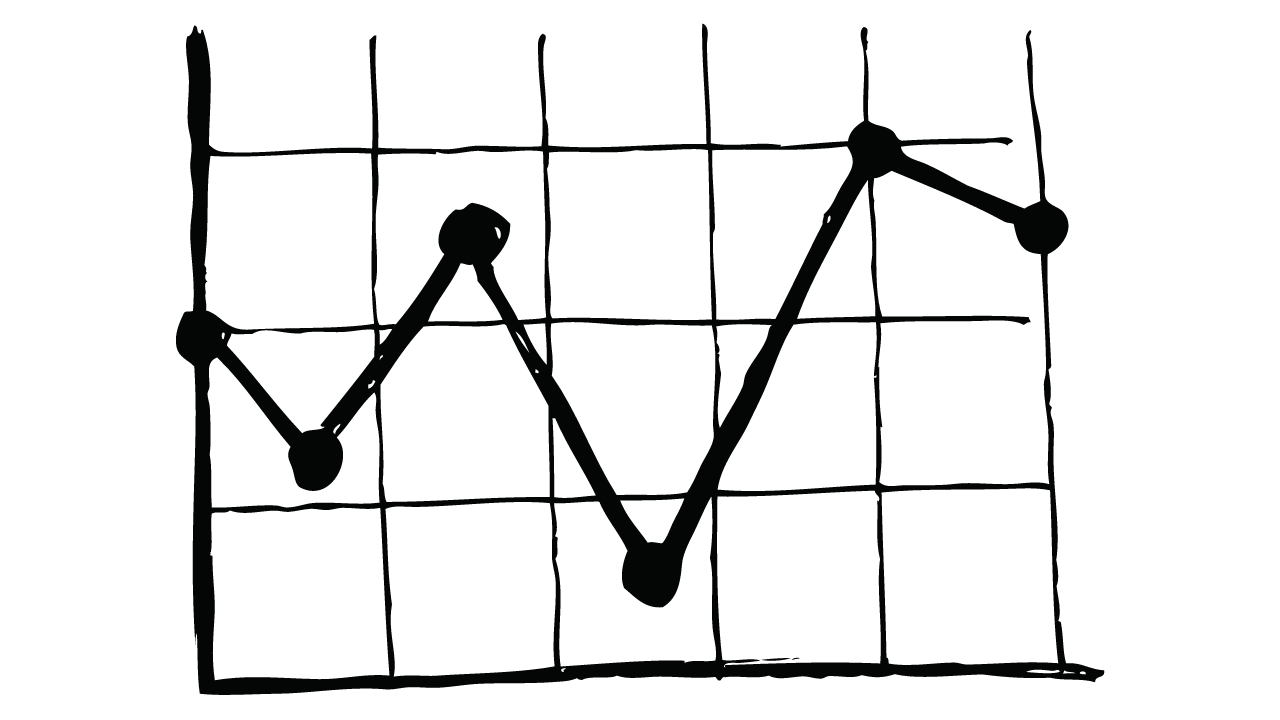
Key Points
- Market Signals
- What are buy sell or hold recommendations?
- What are main components of Markov decision process?
BCM:TSXV Target Price Prediction Modeling Methodology
We consider Bear Creek Mining Corporation Decision Process with Modular Neural Network (Market News Sentiment Analysis) where A is the set of discrete actions of BCM:TSXV stock holders, F is the set of discrete states, P : S × F × S → R is the transition probability distribution, R : S × F → R is the reaction function, and γ ∈ [0, 1] is a move factor for expectation.1,2,3,4
F(Paired T-Test)5,6,7= X R(Modular Neural Network (Market News Sentiment Analysis)) X S(n):→ 1 Year
n:Time series to forecast
p:Price signals of BCM:TSXV stock
j:Nash equilibria (Neural Network)
k:Dominated move
a:Best response for target price
Modular Neural Network (Market News Sentiment Analysis)
A modular neural network (MNN) is a type of artificial neural network that can be used for news feed sentiment analysis. MNNs are made up of multiple smaller neural networks, called modules. Each module is responsible for learning a specific task, such as identifying sentiment in text or identifying patterns in data. The modules are then combined to form a single neural network that can perform multiple tasks. In the context of news feed sentiment analysis, MNNs can be used to identify the sentiment of news articles, social media posts, and other forms of online content. This information can then be used to filter out irrelevant or unwanted content, to identify trends in public opinion, and to target users with relevant advertising.Paired T-Test
A paired t-test is a statistical test that compares the means of two paired samples. In a paired t-test, each data point in one sample is paired with a data point in the other sample. The pairs are typically related in some way, such as before and after measurements, or measurements from the same subject under different conditions. The paired t-test is a parametric test, which means that it assumes that the data is normally distributed. The paired t-test is also a dependent samples test, which means that the data points in each pair are correlated.
For further technical information as per how our model work we invite you to visit the article below:
How do AC Investment Research machine learning (predictive) algorithms actually work?
BCM:TSXV Stock Forecast (Buy or Sell) for 1 Year
Sample Set: Neural NetworkStock/Index: BCM:TSXV Bear Creek Mining Corporation
Time series to forecast n: 17 Jun 2023 for 1 Year
According to price forecasts for 1 Year period, the dominant strategy among neural network is: Buy
X axis: *Likelihood% (The higher the percentage value, the more likely the event will occur.)
Y axis: *Potential Impact% (The higher the percentage value, the more likely the price will deviate.)
Z axis (Grey to Black): *Technical Analysis%
IFRS Reconciliation Adjustments for Bear Creek Mining Corporation
- The following example describes a situation in which an accounting mismatch would be created in profit or loss if the effects of changes in the credit risk of the liability were presented in other comprehensive income. A mortgage bank provides loans to customers and funds those loans by selling bonds with matching characteristics (eg amount outstanding, repayment profile, term and currency) in the market. The contractual terms of the loan permit the mortgage customer to prepay its loan (ie satisfy its obligation to the bank) by buying the corresponding bond at fair value in the market and delivering that bond to the mortgage bank. As a result of that contractual prepayment right, if the credit quality of the bond worsens (and, thus, the fair value of the mortgage bank's liability decreases), the fair value of the mortgage bank's loan asset also decreases. The change in the fair value of the asset reflects the mortgage customer's contractual right to prepay the mortgage loan by buying the underlying bond at fair value (which, in this example, has decreased) and delivering the bond to the mortgage bank. Consequently, the effects of changes in the credit risk of the liability (the bond) will be offset in profit or loss by a corresponding change in the fair value of a financial asset (the loan). If the effects of changes in the liability's credit risk were presented in other comprehensive income there would be an accounting mismatch in profit or loss. Consequently, the mortgage bank is required to present all changes in fair value of the liability (including the effects of changes in the liability's credit risk) in profit or loss.
- Historical information is an important anchor or base from which to measure expected credit losses. However, an entity shall adjust historical data, such as credit loss experience, on the basis of current observable data to reflect the effects of the current conditions and its forecasts of future conditions that did not affect the period on which the historical data is based, and to remove the effects of the conditions in the historical period that are not relevant to the future contractual cash flows. In some cases, the best reasonable and supportable information could be the unadjusted historical information, depending on the nature of the historical information and when it was calculated, compared to circumstances at the reporting date and the characteristics of the financial instrument being considered. Estimates of changes in expected credit losses should reflect, and be directionally consistent with, changes in related observable data from period to period
- If the group of items does not have any offsetting risk positions (for example, a group of foreign currency expenses that affect different line items in the statement of profit or loss and other comprehensive income that are hedged for foreign currency risk) then the reclassified hedging instrument gains or losses shall be apportioned to the line items affected by the hedged items. This apportionment shall be done on a systematic and rational basis and shall not result in the grossing up of the net gains or losses arising from a single hedging instrument.
- An entity need not undertake an exhaustive search for information but shall consider all reasonable and supportable information that is available without undue cost or effort and that is relevant to the estimate of expected credit losses, including the effect of expected prepayments. The information used shall include factors that are specific to the borrower, general economic conditions and an assessment of both the current as well as the forecast direction of conditions at the reporting date. An entity may use various sources of data, that may be both internal (entity-specific) and external. Possible data sources include internal historical credit loss experience, internal ratings, credit loss experience of other entities and external ratings, reports and statistics. Entities that have no, or insufficient, sources of entityspecific data may use peer group experience for the comparable financial instrument (or groups of financial instruments).
*International Financial Reporting Standards (IFRS) adjustment process involves reviewing the company's financial statements and identifying any differences between the company's current accounting practices and the requirements of the IFRS. If there are any such differences, neural network makes adjustments to financial statements to bring them into compliance with the IFRS.
Conclusions
Bear Creek Mining Corporation is assigned short-term Ba1 & long-term Ba1 estimated rating. Bear Creek Mining Corporation prediction model is evaluated with Modular Neural Network (Market News Sentiment Analysis) and Paired T-Test1,2,3,4 and it is concluded that the BCM:TSXV stock is predictable in the short/long term. According to price forecasts for 1 Year period, the dominant strategy among neural network is: Buy
BCM:TSXV Bear Creek Mining Corporation Financial Analysis*
Rating | Short-Term | Long-Term Senior |
---|---|---|
Outlook* | Ba1 | Ba1 |
Income Statement | B3 | B3 |
Balance Sheet | Caa2 | Ba1 |
Leverage Ratios | B1 | Caa2 |
Cash Flow | Caa2 | Baa2 |
Rates of Return and Profitability | Baa2 | Baa2 |
*Financial analysis is the process of evaluating a company's financial performance and position by neural network. It involves reviewing the company's financial statements, including the balance sheet, income statement, and cash flow statement, as well as other financial reports and documents.
How does neural network examine financial reports and understand financial state of the company?
Prediction Confidence Score
References
- V. Borkar and R. Jain. Risk-constrained Markov decision processes. IEEE Transaction on Automatic Control, 2014
- Firth JR. 1957. A synopsis of linguistic theory 1930–1955. In Studies in Linguistic Analysis (Special Volume of the Philological Society), ed. JR Firth, pp. 1–32. Oxford, UK: Blackwell
- Efron B, Hastie T. 2016. Computer Age Statistical Inference, Vol. 5. Cambridge, UK: Cambridge Univ. Press
- Barrett, C. B. (1997), "Heteroscedastic price forecasting for food security management in developing countries," Oxford Development Studies, 25, 225–236.
- Imbens G, Wooldridge J. 2009. Recent developments in the econometrics of program evaluation. J. Econ. Lit. 47:5–86
- Burkov A. 2019. The Hundred-Page Machine Learning Book. Quebec City, Can.: Andriy Burkov
- Hornik K, Stinchcombe M, White H. 1989. Multilayer feedforward networks are universal approximators. Neural Netw. 2:359–66
Frequently Asked Questions
Q: What is the prediction methodology for BCM:TSXV stock?A: BCM:TSXV stock prediction methodology: We evaluate the prediction models Modular Neural Network (Market News Sentiment Analysis) and Paired T-Test
Q: Is BCM:TSXV stock a buy or sell?
A: The dominant strategy among neural network is to Buy BCM:TSXV Stock.
Q: Is Bear Creek Mining Corporation stock a good investment?
A: The consensus rating for Bear Creek Mining Corporation is Buy and is assigned short-term Ba1 & long-term Ba1 estimated rating.
Q: What is the consensus rating of BCM:TSXV stock?
A: The consensus rating for BCM:TSXV is Buy.
Q: What is the prediction period for BCM:TSXV stock?
A: The prediction period for BCM:TSXV is 1 Year