Dominant Strategy : Sell
Time series to forecast n: 13 Jun 2023 for 1 Year
Methodology : Reinforcement Machine Learning (ML)
Abstract
BATTERY MINERALS LIMITED prediction model is evaluated with Reinforcement Machine Learning (ML) and Statistical Hypothesis Testing1,2,3,4 and it is concluded that the BAT stock is predictable in the short/long term. Reinforcement machine learning (RL) is a type of machine learning where an agent learns to take actions in an environment in order to maximize a reward. The agent does this by trial and error, and is able to learn from its mistakes. RL is a powerful tool that can be used for a variety of tasks, including game playing, robotics, and finance. According to price forecasts for 1 Year period, the dominant strategy among neural network is: Sell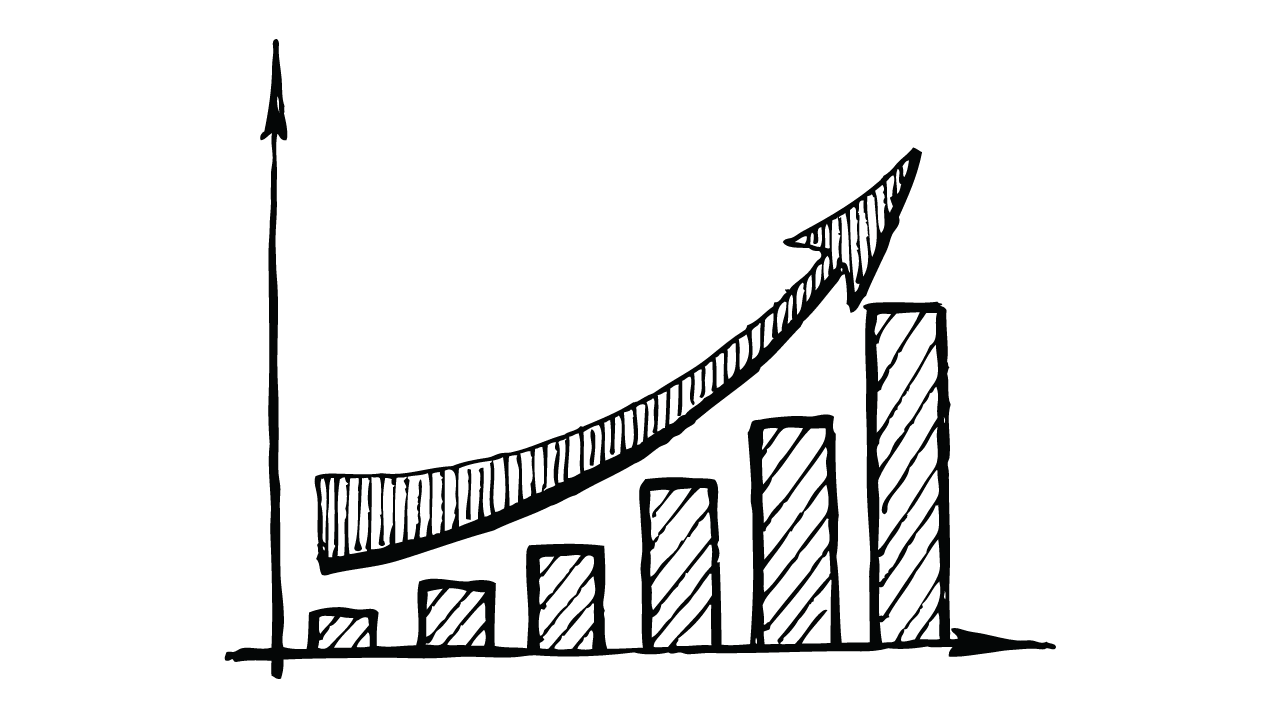
Key Points
- What is prediction in deep learning?
- What are the most successful trading algorithms?
- Prediction Modeling
BAT Target Price Prediction Modeling Methodology
We consider BATTERY MINERALS LIMITED Decision Process with Reinforcement Machine Learning (ML) where A is the set of discrete actions of BAT stock holders, F is the set of discrete states, P : S × F × S → R is the transition probability distribution, R : S × F → R is the reaction function, and γ ∈ [0, 1] is a move factor for expectation.1,2,3,4
F(Statistical Hypothesis Testing)5,6,7= X R(Reinforcement Machine Learning (ML)) X S(n):→ 1 Year
n:Time series to forecast
p:Price signals of BAT stock
j:Nash equilibria (Neural Network)
k:Dominated move
a:Best response for target price
Reinforcement Machine Learning (ML)
Reinforcement machine learning (RL) is a type of machine learning where an agent learns to take actions in an environment in order to maximize a reward. The agent does this by trial and error, and is able to learn from its mistakes. RL is a powerful tool that can be used for a variety of tasks, including game playing, robotics, and finance.Statistical Hypothesis Testing
Statistical hypothesis testing is a process used to determine whether there is enough evidence to support a claim about a population based on a sample. The process involves making two hypotheses, a null hypothesis and an alternative hypothesis, and then collecting data and using statistical tests to determine which hypothesis is more likely to be true. The null hypothesis is the statement that there is no difference between the population and the sample. The alternative hypothesis is the statement that there is a difference between the population and the sample. The statistical test is used to calculate a p-value, which is the probability of obtaining the observed data or more extreme data if the null hypothesis is true. A p-value of less than 0.05 is typically considered to be statistically significant, which means that there is less than a 5% chance of obtaining the observed data or more extreme data if the null hypothesis is true.
For further technical information as per how our model work we invite you to visit the article below:
How do AC Investment Research machine learning (predictive) algorithms actually work?
BAT Stock Forecast (Buy or Sell) for 1 Year
Sample Set: Neural NetworkStock/Index: BAT BATTERY MINERALS LIMITED
Time series to forecast n: 13 Jun 2023 for 1 Year
According to price forecasts for 1 Year period, the dominant strategy among neural network is: Sell
X axis: *Likelihood% (The higher the percentage value, the more likely the event will occur.)
Y axis: *Potential Impact% (The higher the percentage value, the more likely the price will deviate.)
Z axis (Grey to Black): *Technical Analysis%
IFRS Reconciliation Adjustments for BATTERY MINERALS LIMITED
- An entity shall apply this Standard for annual periods beginning on or after 1 January 2018. Earlier application is permitted. If an entity elects to apply this Standard early, it must disclose that fact and apply all of the requirements in this Standard at the same time (but see also paragraphs 7.1.2, 7.2.21 and 7.3.2). It shall also, at the same time, apply the amendments in Appendix C.
- An entity has not retained control of a transferred asset if the transferee has the practical ability to sell the transferred asset. An entity has retained control of a transferred asset if the transferee does not have the practical ability to sell the transferred asset. A transferee has the practical ability to sell the transferred asset if it is traded in an active market because the transferee could repurchase the transferred asset in the market if it needs to return the asset to the entity. For example, a transferee may have the practical ability to sell a transferred asset if the transferred asset is subject to an option that allows the entity to repurchase it, but the transferee can readily obtain the transferred asset in the market if the option is exercised. A transferee does not have the practical ability to sell the transferred asset if the entity retains such an option and the transferee cannot readily obtain the transferred asset in the market if the entity exercises its option
- The significance of a change in the credit risk since initial recognition depends on the risk of a default occurring as at initial recognition. Thus, a given change, in absolute terms, in the risk of a default occurring will be more significant for a financial instrument with a lower initial risk of a default occurring compared to a financial instrument with a higher initial risk of a default occurring.
- There is a rebuttable presumption that unless inflation risk is contractually specified, it is not separately identifiable and reliably measurable and hence cannot be designated as a risk component of a financial instrument. However, in limited cases, it is possible to identify a risk component for inflation risk that is separately identifiable and reliably measurable because of the particular circumstances of the inflation environment and the relevant debt market
*International Financial Reporting Standards (IFRS) adjustment process involves reviewing the company's financial statements and identifying any differences between the company's current accounting practices and the requirements of the IFRS. If there are any such differences, neural network makes adjustments to financial statements to bring them into compliance with the IFRS.
Conclusions
BATTERY MINERALS LIMITED is assigned short-term Ba1 & long-term Ba1 estimated rating. BATTERY MINERALS LIMITED prediction model is evaluated with Reinforcement Machine Learning (ML) and Statistical Hypothesis Testing1,2,3,4 and it is concluded that the BAT stock is predictable in the short/long term. According to price forecasts for 1 Year period, the dominant strategy among neural network is: Sell
BAT BATTERY MINERALS LIMITED Financial Analysis*
Rating | Short-Term | Long-Term Senior |
---|---|---|
Outlook* | Ba1 | Ba1 |
Income Statement | B3 | Baa2 |
Balance Sheet | Caa2 | B1 |
Leverage Ratios | Ba1 | B3 |
Cash Flow | C | Baa2 |
Rates of Return and Profitability | Caa2 | Baa2 |
*Financial analysis is the process of evaluating a company's financial performance and position by neural network. It involves reviewing the company's financial statements, including the balance sheet, income statement, and cash flow statement, as well as other financial reports and documents.
How does neural network examine financial reports and understand financial state of the company?
Prediction Confidence Score
References
- Hoerl AE, Kennard RW. 1970. Ridge regression: biased estimation for nonorthogonal problems. Technometrics 12:55–67
- Abadie A, Cattaneo MD. 2018. Econometric methods for program evaluation. Annu. Rev. Econ. 10:465–503
- Firth JR. 1957. A synopsis of linguistic theory 1930–1955. In Studies in Linguistic Analysis (Special Volume of the Philological Society), ed. JR Firth, pp. 1–32. Oxford, UK: Blackwell
- M. Ono, M. Pavone, Y. Kuwata, and J. Balaram. Chance-constrained dynamic programming with application to risk-aware robotic space exploration. Autonomous Robots, 39(4):555–571, 2015
- Hoerl AE, Kennard RW. 1970. Ridge regression: biased estimation for nonorthogonal problems. Technometrics 12:55–67
- K. Tuyls and G. Weiss. Multiagent learning: Basics, challenges, and prospects. AI Magazine, 33(3): 41–52, 2012
- Bai J, Ng S. 2017. Principal components and regularized estimation of factor models. arXiv:1708.08137 [stat.ME]
Frequently Asked Questions
Q: What is the prediction methodology for BAT stock?A: BAT stock prediction methodology: We evaluate the prediction models Reinforcement Machine Learning (ML) and Statistical Hypothesis Testing
Q: Is BAT stock a buy or sell?
A: The dominant strategy among neural network is to Sell BAT Stock.
Q: Is BATTERY MINERALS LIMITED stock a good investment?
A: The consensus rating for BATTERY MINERALS LIMITED is Sell and is assigned short-term Ba1 & long-term Ba1 estimated rating.
Q: What is the consensus rating of BAT stock?
A: The consensus rating for BAT is Sell.
Q: What is the prediction period for BAT stock?
A: The prediction period for BAT is 1 Year